New Enzymes from Heme Proteins
Nature’s heme-binding proteins perform a remarkably diverse set of biological functions, from gas and electron transport to roles in plant-animal interactions. While evolution optimized these proteins for their native roles, many also exhibit “promiscuous” activities—functions not used in their natural contexts but with potential for further functional evolution. Leveraging this natural diversity, we use directed evolution to transform heme proteins into enzymes capable of catalyzing non-biological reactions with high efficiency and selectivity.
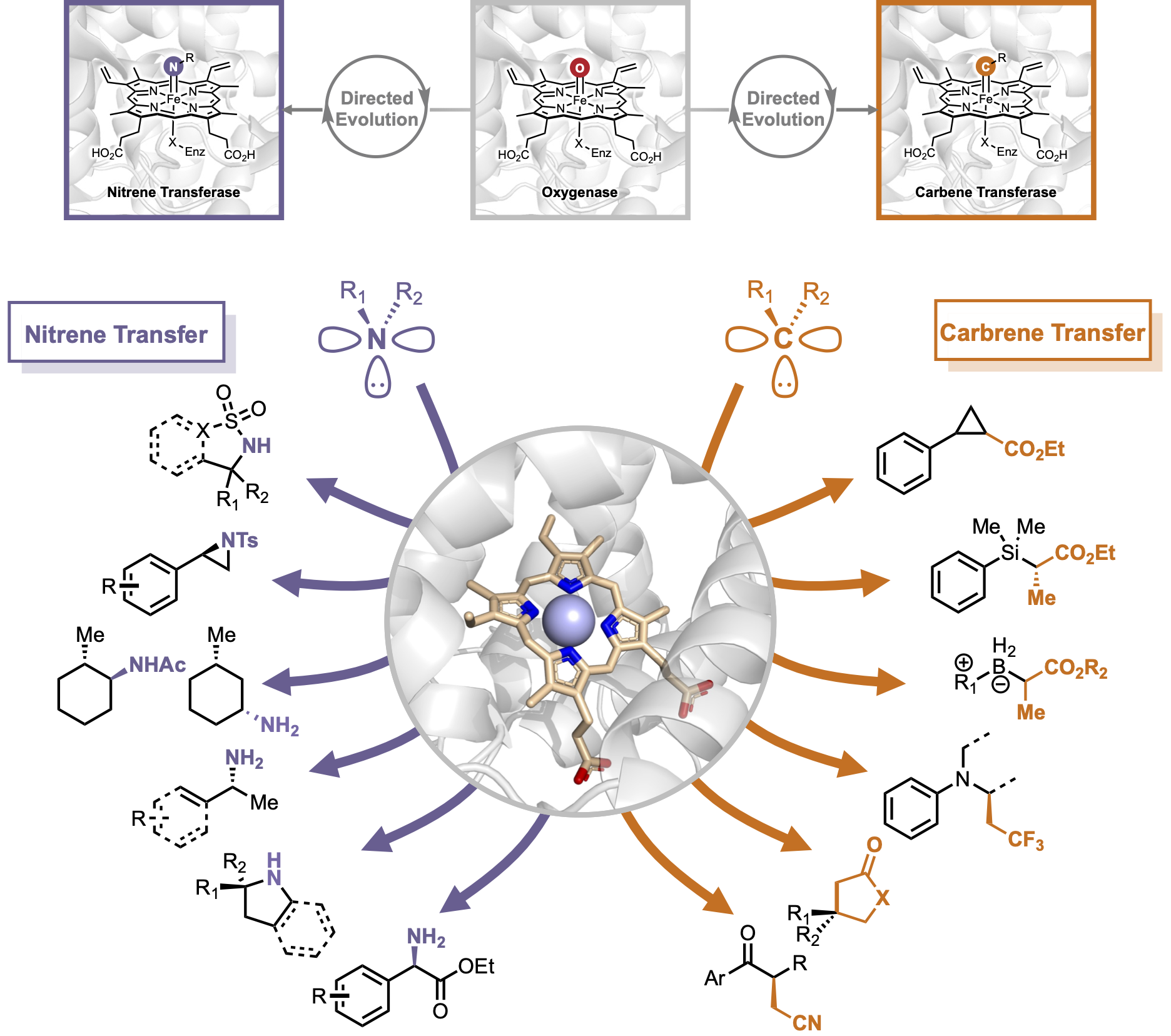
Nitrene Transfer
A transition-metal nitrenoid is a key reactive intermediate for constructing carbon–nitrogen bonds, essential for synthesizing nitrogen-containing functional molecules. While nitrene transfer reactions are widely used in synthetic chemistry, their occurrence in biological systems was only recently discovered. Traditionally, chemists have relied on expensive and unsustainable transition-metal catalysts, such as rhodium (Rh), ruthenium (Ru), and iridium (Ir), for stereoselective nitrene transfer. In contrast, our enzymes utilize iron—an earth-abundant, environmentally benign metal—offering a more sustainable approach to carbon–nitrogen bond formation.
We have engineered a variety of heme-dependent enzymes, including cytochromes P450, cytochromes c, and globins, to perform diverse asymmetric nitrene transfer reactions. These include aziridination, alkene aminohydroxylation, and C–H amination, facilitating the efficient synthesis of chiral amines, N-heterocycles, and non-canonical amino acids. The catalytic activities and selectivities of these genetically encoded enzymes can be further optimized through directed evolution, achieving unprecedented levels of reactivity and chemo-, regio-, and stereoselectivity.
Some Papers to Start with:
“Biocatalytic Synthesis of α-Amino Esters via Nitrene C–H Insertion” E. Alfonzo, D. Hanley, Z.-Q. Li, K. M. Sicinski, S. Gao, and F. H. Arnold. J. Am. Chem. Soc. September 27, 2024. 10.1021/jacs.4c09989. “Reaction Discovery Using Spectroscopic Insights from an Enzymatic C–H Amination Intermediate” A. Das, S. Gao, R. G. Lal, M. H. Hicks, P. H. Oyala, and F. H. Arnold. J. Am. Chem. Soc. July 22, 2024. 10.1021/jacs.4c05761. “Biocatalytic Construction of Chiral Pyrrolidines and Indolines via Intramolecular C(sp3)–H Amination” Z-Y. Qin, S. Gao, Y. Zou, Z. Liu, J. B. Wang, K. N. Houk, and F. H. Arnold. ACS Cent. Sci. December 14, 2023. 10.1021/acscentsci.3c00516. “Enzymatic Nitrogen Incorporation Using Hydroxylamine” S. Gao, A. Das, E. Alfonzo, K. M. Sicinski, D. Rieger, and F. H. Arnold. J. Am. Chem. Soc. September 6, 2023. 10.1021/jacs.3c08053. “Enzymatic Nitrogen Insertion into Unactivated C–H bonds” S. V. Athavale†, S. Gao†, A. Das†, S. C. Mallojjala, E. Alfonzo, Y. Long, J. S. Hirschi, and F. H. Arnold. J. Am. Chem. Soc. October 4, 2022. 10.1021/jacs.2c08285. Perspective by Derek Lowe: Zapping in Amine Groups. Synfacts highlight: Enzymatic Amination and Amidation of Unactivated Csp3–H Sites.Carbene Transfer
The formation of new carbon–carbon bonds is fundamental to novel compound discovery and chemical manufacturing. However, achieving high chemo-, regio-, and stereoselectivity in chemical synthesis remains a significant challenge. Our lab harnesses the ability of hemoproteins to generate reactive iron-carbenoid intermediates for constructing carbon–carbon bonds through carbene transfer reactions. By applying directed evolution, we engineer these biocatalysts to achieve activity and selectivity that often surpasses traditional synthetic catalysts.
We have successfully reprogrammed various heme proteins, including cytochromes P450, cytochromes c, myoglobins, hemoglobins, and protoglobins, to perform carbon–carbon bond forming reactions that are not known to occur in biological systems. Examples include cyclopropanation, cyclopropenation, bicyclobutanation, and even carbene C–H insertion. Through directed evolution, we have expanded the repertoire of both compatible carbene species and functional groups in our enzymatic platform. Notably, we evolved the first biocatalysts capable of forming C–Si and C–B bonds, further broadening the scope of enzymatic transformations.
Some Papers to Start with:
“Enzymatic Assembly of Diverse Lactone Structures: An Intramolecular C–H Functionalization Strategy” D. J. Wackelin, R. Mao, K. M. Sicinski, Y. Zhao, A. Das, K. Chen, and F. H. Arnold. J. Am. Chem. Soc. January 2, 2024. 10.1021/jacs.3c11722. “Biocatalytic, stereoconvergent alkylation of (Z/E)-trisubstituted silyl enol ethers” R. Mao, D. M. Taylor, D. J. Wackelin, T. Rogge, S. J. Wu, K. M. Sicinski, K. N. Houk, and F. H. Arnold. Nat. Synth. November 2, 2023. 10.1038/s44160-023-00431-2. “Chemodivergent C(sp3)–H and C(sp2)–H cyanomethylation using engineered carbene transferases” J. Zhang, A. O. Maggiolo, E. Alfonzo, R. Mao, N. J. Porter, N. M. Abney, and F. H. Arnold. Nat. Catal. January 19, 2023. 10.1038/s41929-022-00908-x. “Biocatalytic Carbene Transfer Using Diazirines” N. J. Porter, E. Danelius, T. Gonen, & F. H. Arnold. J. Am. Chem. Soc. May 13, 2022. 10.1021/jacs.2c02723. “Enzymatic construction of highly strained carbocycles” K. Chen, X. Huang, S. B. J. Kan, R. K. Zhang, F. H. Arnold. Science. April 6, 2018. 10.1126/science.aar4239.New Uses for Tryptophan Synthase
We believe that enzymes can tackle some of the biggest challenges in synthetic chemistry. Recently we have begun to engineer enzymes to produce valuable building blocks for the synthesis of bioactive compounds. Noncanonical amino acids (ncAAs) are important components of natural and artificial products; they are also useful tools for chemical biology. Enzymes can produce ncAAs with pristine enantioselectivity, without the need for protecting groups or expensive reagents.
To create a scalable enzymatic platform for synthesis of Trp analogs, we identified a TrpB subunit of the tryptophan synthase (TrpS) complex and engineered it to function as an independent enzyme.
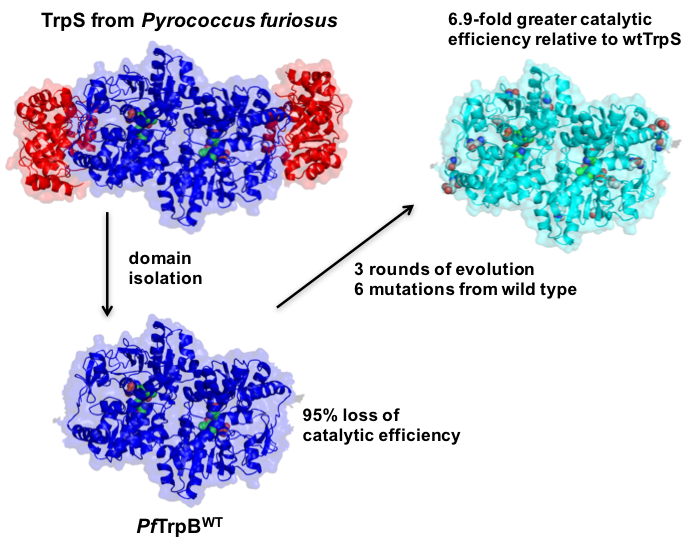
This stand-alone enzyme has served as the basis for engineering a panel of catalysts that have been used to make more than 70 different ncAAs (and counting)!
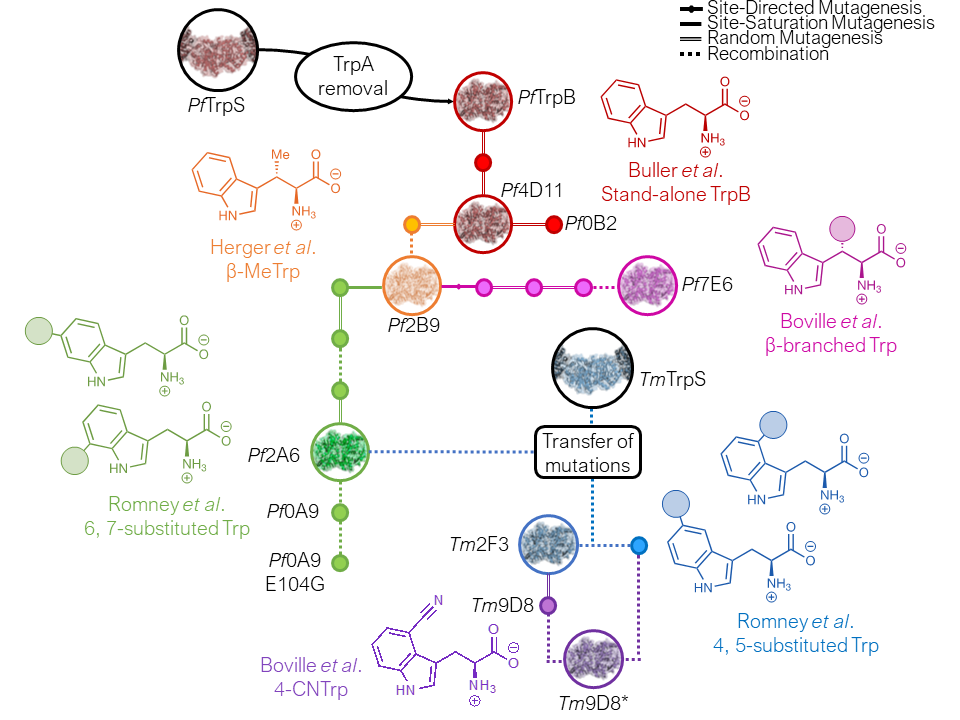
We are now expanding the substrate scope of TrpB to create a general platform for synthesis of noncanonical amino acids. Recent advances include the engineering of TrpB to produce a diverse repertoire of tryptophan analogs, as well as its functional repurposing into tyrosine synthases.
Some Papers to Start with:
“The β-subunit of tryptophan synthase is a latent tyrosine synthase“ P. J. Almhjell, K. E. Johnston, N. J. Porter, J. L. Kennemur, V. C. Bhethanabotla, J. Ducharme, and F. H. Arnold. Nat. Chem. Biol. May 14, 2024. 10.1038/s41589-024-01619-z.
“Tailoring tryptophan synthase for selective quaternary carbon bond formation“ M. Dick, N. S. Sarai, M. W. Martynowycz, T. Gonen, F. H. Arnold. . J. Am. Chem. Soc. November 20, 2019. 10.1021/jacs.9b09864.
“Engineering enzymes for noncanonical amino acid synthesis“ P. J. Almhjell, C. E. Boville, F. H. Arnold. Chem. Soc. Rev. December 21, 2018. 10.1039/C8CS00665B.
“Engineered biosynthesis of β‐alkyl tryptophan analogs“ C. E. Boville, R. A. Scheele, P. Koch, S. Brinkmann-Chen, A. R. Buller, F. H. Arnold. Angew. Chem., September 14, 2018. 10.1002/anie.201807998
“Unlocking reactivity of TrpB: A general biocatalytic platform for synthesis of tryptophan analogues” D. K. Romney, J. Murciano-Calles, J. E. Wehrmuller, F. H. Arnold. J. Am. Chem. Soc., July 14, 2017. 10.1021/jacs.7b05007.
“Directed evolution of the tryptophan synthase β-subunit for stand-alone function recapitulates allosteric activation” A. R. Buller, S. Brinkmann-Chen, D. K. Romney, M. Herger, J. Murciano-Calles, F. H. Arnold. PNAS. , November 9, 2015. 10.1073/pnas.1516401112.
Computational Tools for Protein Engineering
Overview
The Arnold laboratory has developed and implemented various computational tools to advance protein engineering, with a particular interested in enzyme engineering. Our toolbox encompasses identifying starting points for enzyme engineering, optimizing the engineering process through machine learning, and creating in-house sequencing tools to streamline data collection and analysis.
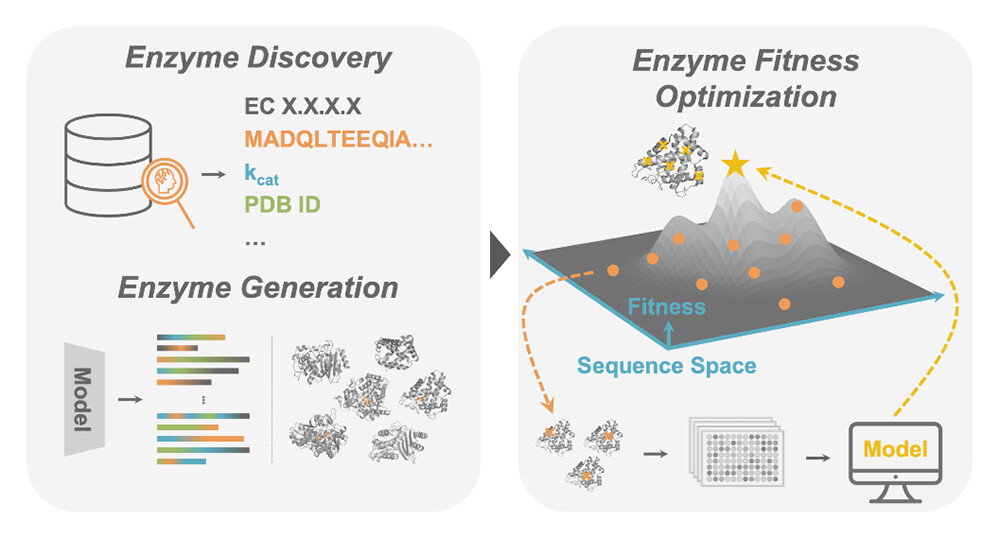
Some Papers to Start with:
“Opportunities and Challenges for Machine Learning-Assisted Enzyme Engineering” J. Yang, F.-Z. Li, and F. H. Arnold. ACS Cent. Sci. February 5, 2024. 10.1021/acscentsci.3c01275.
Computational Methods to Identify Starting Points for Engineering New Enzymatic Activities
Most directed evolution campaigns for new activities (reactions) require an initial starting point: an existing protein with some promiscuous activity for the desired new activity. However, identifying such starting points can be highly challenging. While trace activity for certain new reactions can be found by screening existing evolved variants, other more difficult activities may remain elusive. In these cases, experimentalists may need to perform a laborious search through public databases and screen proteins with similar cofactors, active sites, or catalytic mechanisms. This process requires significant chemical intuition and expertise in structural biology, making it an arduous task.
To address this challenge, our lab developed CREEP, a computational workflow designed to identify enzymes with unannotated reactivities. The CREEP model is a contrastive learning model that can retrieve enzyme sequences for target reactions that are unseen by the model. While CREEP has demonstrated solid performance in computational baselines, there is still room for improvement and for wet-lab validation. The challenge of reliably finding starting points remains largely unsolved.
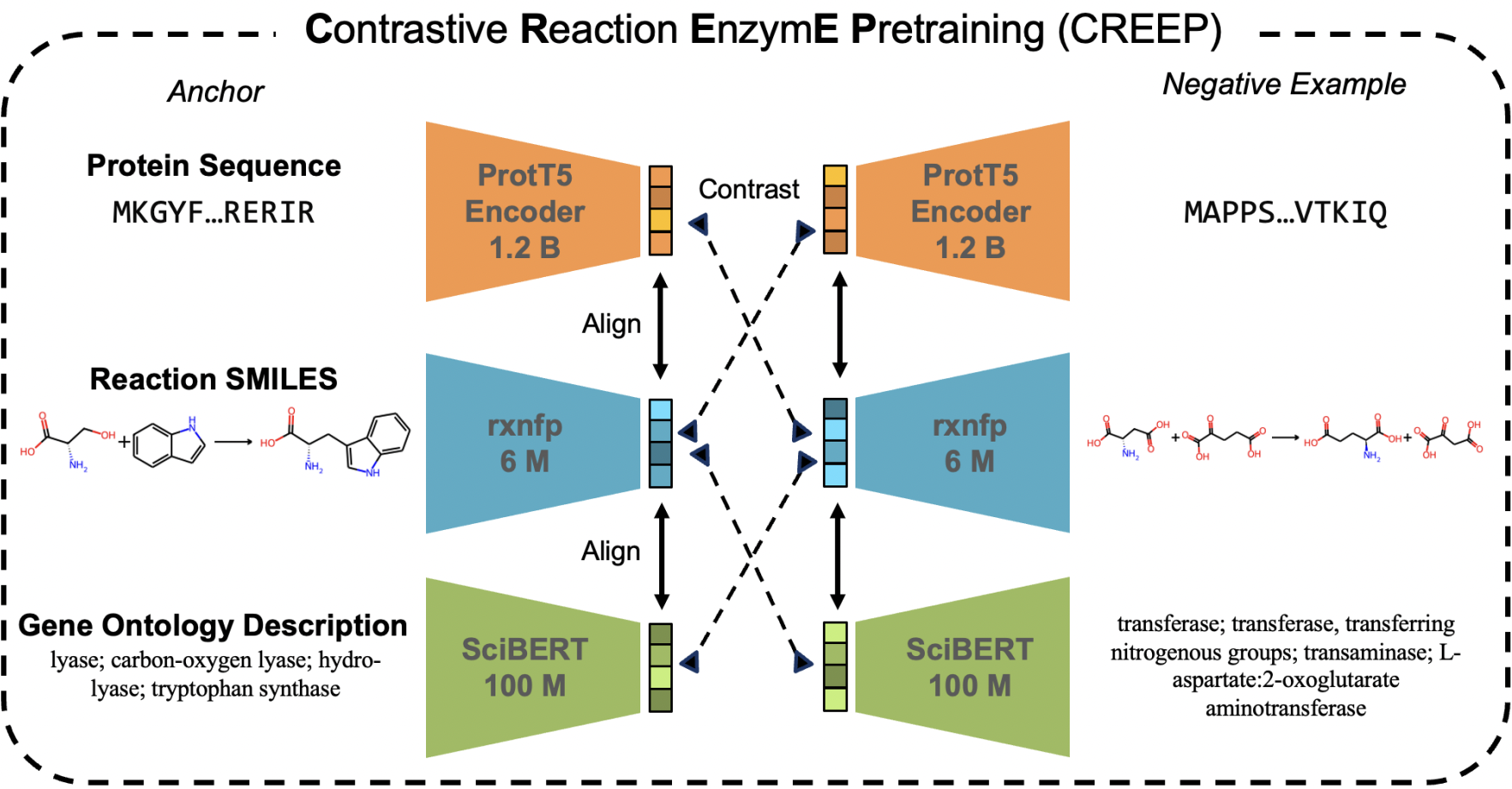
Some Papers to Start with:
“CARE: a Benchmark Suite for the Classification and Retrieval of Enzymes” J. Yang, A. Mora, S. Liu, B. J. Wittmann, A. Anandkumar, F. H. Arnold, and Y. Yue. aRxiv. June 21, 2024. 2406.15669.
Machine Learning-Assisted Directed Evolution
Despite its widespread use, directed evolution remains time-consuming and resource-intensive. Screening is expensive, and multiple rounds of mutation and screening are required to achieve desired improvements. Additionally, epistasis—the non-additive interactions between amino acid substitutions—poses significant challenges. For example, mutations beneficial in an initial sequence may lose their advantage when combined with other changes.
Machine learning-assisted directed evolution (MLDE) addresses these limitations by learning the relationship between protein sequences and fitness (numerical metrics for protein function) from small training (experimental) datasets to predict high-fitness variants across the sequence landscape. Building on our original MLDE framework, we introduced two strategies: active learning-assisted directed evolution (ALDE) and focused training. ALDE is an iterative MLDE approach where each successive round of sampling is guided by the uncertainty quantified in the previous iteration. Focused-training uses zero-shot (ZS) predictors to create a more informative initial training set. ZS predictors estimate protein fitness without the need for experimental data: they are instead based on prior assumptions and leverage auxiliary information, such as protein stability calculations, evolutionary data, or structural information.
Most recently, we evaluated ALDE in the wet lab and tested various MLDE strategies computationally over 16 diverse protein-fitness landscapes. These efforts demonstrated the broad utility of MLDE and initiated the establishment of best practices for using it. Current research involves improving these MLDE workflows in the wet-lab and leveraging generative models for more efficient fitness optimization over large design spaces.
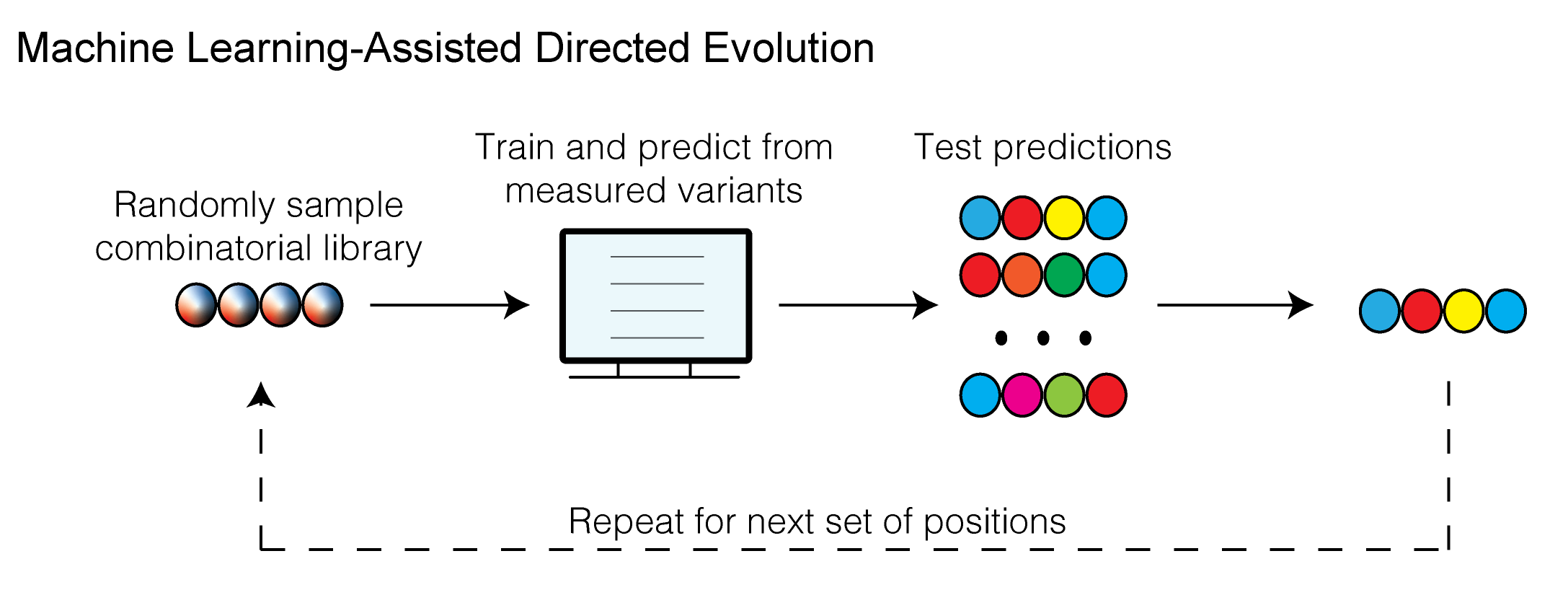
Some Papers to Start with:
“Machine-learning-guided directed evolution for protein engineering” K. Yang, Z. Wu, F. H. Arnold. Nature Methods. July 15, 2019. 10.1038/s41592-019-0496-6.
“Machine-Learning-Assisted Directed Protein Evolution with Combinatorial Libraries” Z. Wu, S. B. J. Kan, R. D. Lewis, B. J. Wittmann, F. H. Arnold. PNAS, April 12, 2019. 10.1073/pnas.1901979116.
“Informed training set design enables efficient machine learning-assisted directed protein evolution” B. J. Wittmann, Y. Yue, & F. H. Arnold. Cell Syst, August 19, 2021. 10.1016/j.cels.2021.07.008.
“Active Learning-Assisted Directed Evolution” J. Yang, R. G. Lal, J. C. Bowden, R. Astudillo, M. A. Hameedi, S. Kaur, M. Hill, Y. Yue, and F. H. Arnold. Nat. Commun, January 16, 2025. 10.1038/s41467-025-55987-8.
“Evaluation of Machine Learning-Assisted Directed Evolution Across Diverse Combinatorial Landscapes” F.-Z. Li, J. Yang, K. E. Johnston, E. Gürsoy, Y. Yue, and F. H. Arnold.. biorxiv, October 24, 2024. 10.1101/2024.10.24.619774.
Sequencing Technology Development to Enable Data-Driven Workflows and Dataset Generation to Benchmark Computational Tools
Our lab also develops advanced sequencing technologies to facilitate the collection of protein sequence-fitness data and generates comprehensive datasets designed to benchmark computational tools effectively.
Sequencing Technology Development
Our lab is committed to bridging computational workflows with real-world demonstrations. ML-assisted workflows require substantial and quality labeled data—pairs of sequences and their associated fitness values. However, collecting this data can be challenging since many directed evolution workflows do not typically generate sequence data (only fitness). Moreover, sequencing many individual protein variants can be prohibitively expensive.
To overcome this challenge, we developed evSeq (every variant Sequencing) and LevSeq (Long-read every variant Sequencing), methods that use pooling and barcoding to cost-effectively sequence all protein variants from directed evolution campaigns in 96-well plates using Illumina and nanopore sequencing, respectively. Both evSeq and LevSeq are accompanied by wet-lab protocols and computational analysis pipelines, making them easy-to-use and significantly reducing the sequencing cost.
With these tools, we are now able to collect hundreds of sequence-fitness pairs for each directed evolution campaign! We have also used data collected from LevSeq in wet-lab demonstrations of MLDE. However, ensuring this data is usable for ML models and other applications remains a priority. As such, our lab is actively working on developing a standardized database to store and organize this information in a practical and efficient manner.
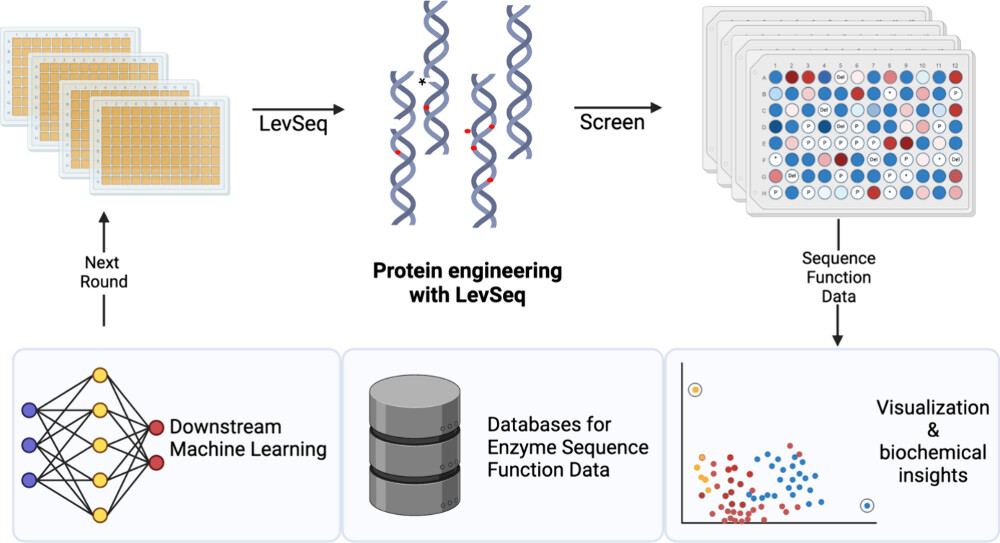
Some Papers to Start with:
“LevSeq: Rapid Generation of Sequence-Function Data for Directed Evolution and Machine Learning” Y. Long, A. Mora, F.-Z. Li, E. Gürsoy, K. E. Johnston, and F. H. Arnold. ACS Synth. Biol. December 24, 2024. 10.1021/acssynbio.4c00625.
“evSeq: Cost-Effective Amplicon Sequencing of Every Variant in a Protein Library” B. J. Wittmann, K. E. Johnston, P. J. Almhjell, and F. H. Arnold. ACS Synth. Biol. February 17, 2022. 10.1021/acssynbio.1c00592.
New Datasets
Comprehensive datasets are essential for understanding the intricate relationship between protein sequences and fitness values, as well as for empowering machine learning models to accelerate protein engineering. However, most existing datasets focus on the effects of single substitutions across diverse protein functions, leaving the more challenging multi-substitution interactions largely unaddressed. Even rarer are datasets that thoroughly analyze these interactions in the context of catalytic functions.
To address this gap, our lab constructed and analyzed a 160,000-member enzyme sequence-fitness dataset for tryptophan synthase. This dataset captures the interactions of substitutions at four residues near the enzyme’s active site, revealing significant non-additive effects on catalytic function.
Building on this foundation, we are actively generating new datasets that expand coverage of active site residues. These efforts aim to deepen our understanding of enzyme active site engineering and provide invaluable resources for optimizing directed evolution workflows.
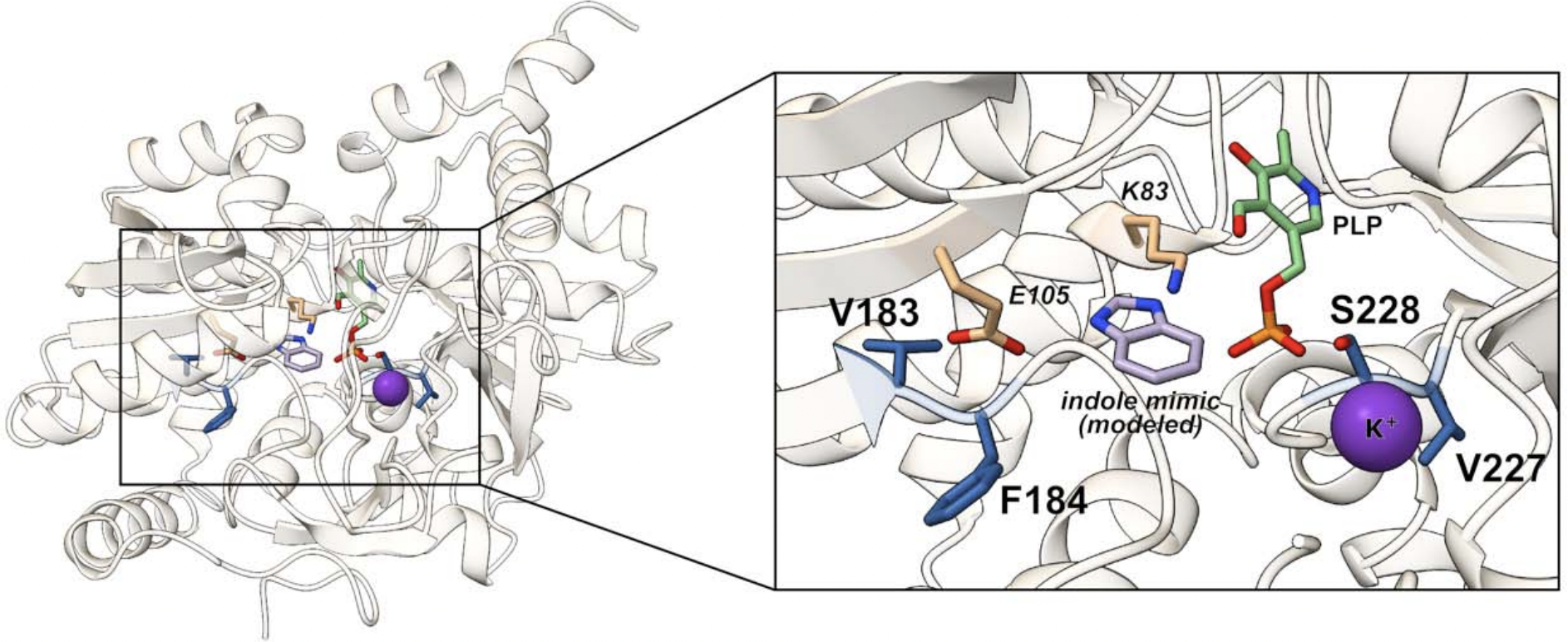
Some Papers to Start with:
“A combinatorially complete epistatic fitness landscape in an enzyme active site” K. E. Johnston, P. J. Almhjell, E. J. Watkins-Dulaney, G. Liu, N. J. Porter, J. Yang, and F. H. Arnold. PNAS. July 29, 2024. 10.1073/pnas.2400439121.